Act
When operating in the real world, with and alongside humans, the ability to re-use skills learnt previously as well as the ability to safely and efficiently acquire new skills is essential. The advent of deep learning has, in recent years, significantly advanced the state-of-the-art with systems which learn end-to-end, reactive control policies directly based on sensor data. These, however, require an often infeasible amount of labelled data – or task demonstrations – to be usable in an agile, collaborative context. This theme will endow robots with an ability to learn new skills based on a minimum of cross-modal user demonstration or by safely training within a learned and curated worldmodel of the environment. Skills so acquired will be re-usable due to versatile goal-conditioning and honed in-situ via active learning.
Mitchell AL, Merkt W, Geisert M, Gangapurwala S, Engelcke M, Parker Jones O, Havoutis I, Posner I
2022 International Conference on Robotics and Automation (ICRA)
Quadruped locomotion is rapidly maturing to a degree where robots now routinely traverse a variety of unstructured terrains. However, while gaits can be varied typically by selecting from a range of pre-computed styles, current planners are unable to vary key gait parameters continuously while the robot is in motion.
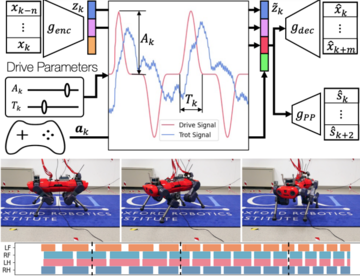
The synthesis, on-the-fly, of gaits with unexpected operational characteristics or even the blending of dynamic manoeuvres lies beyond the capabilities of the current state-of-the-art. In this work we address this limitation by learning a latent space capturing the key stance phases of a particular gait, via a generative model trained on a single trot style. This encourages disentanglement such that application of a drive signal to a single dimension of the latent state induces holistic plans synthesising a continuous variety of trot styles. In fact properties of this drive signal map directly to gait parameters such as cadence, footstep height and full stance duration. The use of a generative model facilitates the detection and mitigation of disturbances to provide a versatile and robust planning framework. We evaluate our approach on a real ANYmal quadruped robot and demonstrate that our method achieves a continuous blend of dynamic trot styles whilst being robust and reactive to external perturbations.
Yamada J, Hung CM, Collins J, Havoutis I, Posner I
IEEE International Conference on Robotics and Automation (ICRA), 2023
Motion planning framed as optimisation in structured latent spaces has recently emerged as competitive with traditional methods in terms of planning success while significantly outperforming them in terms of computational speed. However, the real-world applicability of recent work in this domain remains limited by the need to express obstacle information directly in state-space, involving simple geometric primitives.
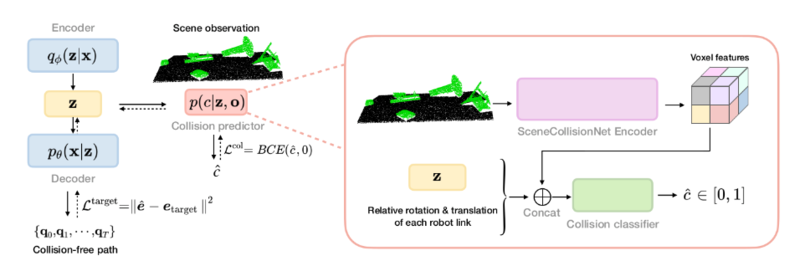
In this work we address this challenge by leveraging learned scene embeddings together with a generative model of the robot manipulator to drive the optimisation process. In addition, we introduce an approach for efficient collision checking which directly regularises the optimisation undertaken for planning. Using simulated as well as real-world experiments, we demonstrate that our approach, AMP-LS, is able to successfully plan in novel, complex scenes while outperforming traditional planning baselines in terms of computation speed by an order of magnitude. We show that the resulting system is fast enough to enable closed-loop planning in real-world dynamic scenes.