Interpret
Abstract, symbolic scene representations lend themselves naturally to the specification and execution of complex tasks and provide the substrate for effective human-machine collaboration. Competencies such as object detection in vision and 3D are well established and commonly leveraged for these tasks. However, their application for wide-scale robot deployment particularly in our Flagship domains is significantly impeded by the lack of available labelled training data. This theme will focus on methods which drive the required supervisory input to zero. It will develop methods for weakly- and unsupervised learning at scale and across multiple modalities such as vision, 3D, and tactile. In drawing innovative parallels with human metacognition it will cast a novel light on safe, robust and efficient autonomy.
Goodwin W, Vaze S, Havoutis I, Posner I
Object pose estimation is an important component of most vision pipelines for embodied agents, as well as in 3D vision more generally. In this paper we tackle the problem of estimating the pose of novel object categories in a zero-shot manner.
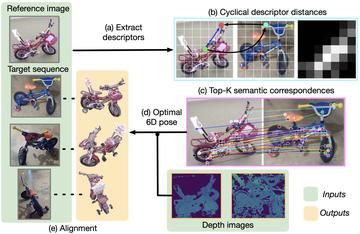
This extends much of the existing literature by removing the need for pose-labelled datasets or category-specific CAD models for training or inference. Specifically, we make the following contributions. First, we formalise the zero-shot, category-level pose estimation problem and frame it in a way that is most applicable to real-world embodied agents. Secondly, we propose a novel method based on semantic correspondences from a self-supervised vision transformer to solve the pose estimation problem. We further re-purpose the recent CO3D dataset to present a controlled and realistic test setting. Finally, we demonstrate that all baselines for our proposed task perform poorly, and show that our method provides a six-fold improvement in rotation estimation accuracy.
Zhong S, Albini A, Parker Jones OP, Maiolino P, Posner I
Tactile perception is key to robotics applications such as manipulation and exploration. However, collecting tactile data is time-consuming, especially when compared to visual data acquisition. This constraint limits the use of tactile data in machine learning solutions to robotics applications. In this paper, we propose a generative model to simulate realistic tactile sensory data for use in downstream robotics tasks.
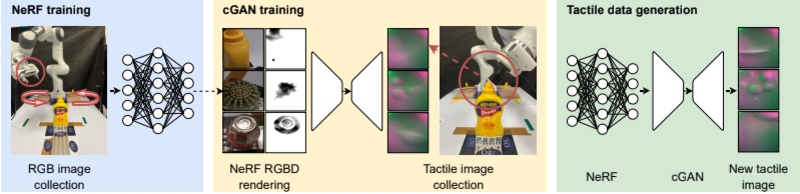
The proposed generative model takes RGB-D images as inputs, and generates corresponding tactile images. The experimental results demonstrate the potential for the proposed approach to generate realistic tactile sensory data and augment manually collected tactile datasets. In addition, we demonstrate that our model is able to transfer from one tactile sensor to another with a small fine-tuning dataset.